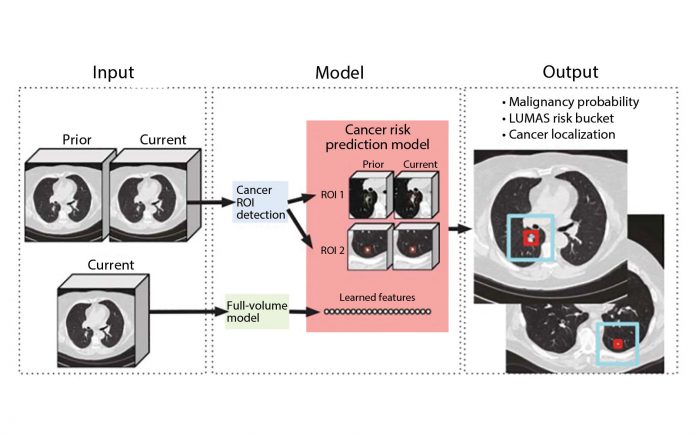
For each patient, the model uses a primary low-dose CT (LDCT) volume and, if available, a prior LDCT volume as input. The model then analyzes suspicious and volumetric ROIs as well as the whole-LDCT volume and outputs an overall malignancy prediction for the case, a risk bucket score (LUMAS), and localization for predicted cancerous nodules.
Reprinted by permission from Springer Nature: Springer Nature, Nature Medicine, End-to-end lung cancer screening with three-dimensional deep learning on low-dose chest computed tomography, Diego Ardila et al, 2019.
By Mizuki Nishino, MD, MPH
Posted: April 16, 2020
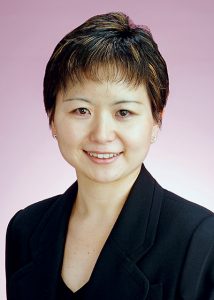
Artificial intelligence (AI) approaches have emerged as promising tools to address important unmet needs across different specialties in medicine, including oncology, radiology, and pathology. Recent studies have described the utility of AI in lung cancer to improve detection, diagnosis, and prognostication. Many of the AI studies in lung cancer involve computational analyses of imaging data, most commonly CT scans, to achieve better outcomes. The detailed analyses of imaging-derived information can be particularly beneficial to facilitate detection and improve diagnostic accuracy of lung cancer screening, as delineated in the recent article, “End-to-end lung cancer screening with three-dimensional deep learning on low-dose chest computed tomography,” by Ardila et al., published in Nature Medicine.1
In this study, Ardila et al. aimed to build an end-to-end approach that performs both lesion localization and lung cancer risk categorization based on the input CT data, in order to simulate a complete workflow of radiologists for lung cancer CT screening. The workflow included a full assessment of CT volume, region-of-interest detection, comparison to prior imaging, and correlation with biopsy-derived results (Fig 1).1 The “end-to-end” design is particularly important when considering AI application in lung cancer because the human workflow consists of multiple steps, and these steps can interact with each other, which adds further complexity to the process. Replicating the entire workflow using the AI model, although challenging, has the potential to provide more robust solutions to improve efficiency and performance of lung cancer CT screening, rather than providing separate tools for individual steps.
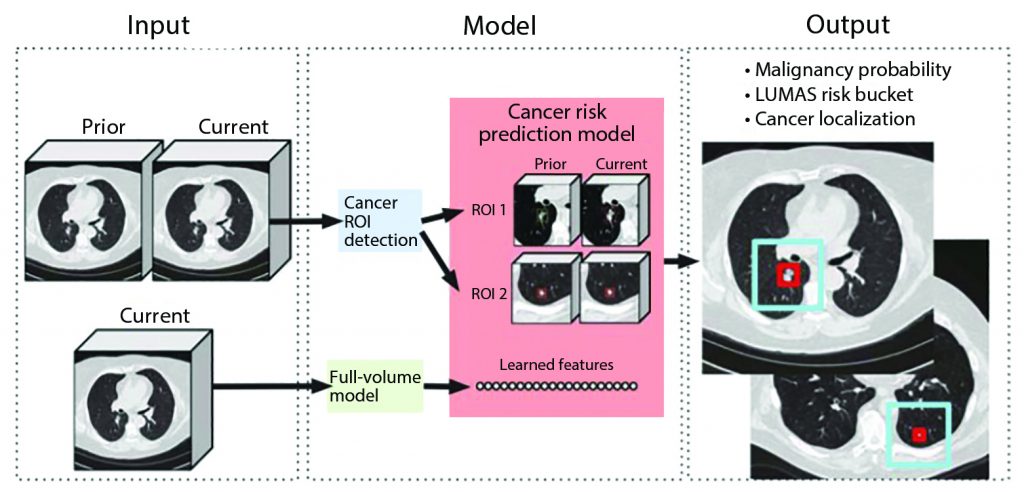
For each patient, the model uses a primary low-dose CT (LDCT) volume and, if available, a prior LDCT volume as input. The model then analyzes suspicious and volumetric ROIs as well as the whole-LDCT volume and outputs an overall malignancy prediction for the case, a risk bucket score (LUMAS), and localization for predicted cancerous nodules.
Reprinted by permission from Springer Nature: Springer Nature, Nature Medicine, End-to-end lung cancer screening with three-dimensional deep learning on low-dose chest computed tomography, Diego Ardila et al, 2019.
The models in the study were built using deep convolutional neural networks (CNN), a powerful way to learn useful representations of images and other structured data, which formed a basis of the current trends of the AI application in medical imaging.2 Using CNN, the models can learn useful features automatically and directly from the raw data, which has been shown to be superior to hand-engineered features.1,2 Using the state-of-the-art AI approaches, the authors built a three-dimensional (3D) CNN model for the whole-CT volume analyses using screening CT.1 Then, a model for CNN region-of-interest (ROI) detection was trained to detect 3D cancer candidate regions in the CT volume. Finally, a CNN cancer risk-prediction model was developed, which operated on the outputs from the other two models (Fig 2).1 The risk prediction model can also incorporate regions of interest from the prior scans by assessing the regions corresponding to the cancer candidate regions on the current scan. This particular feature of the model integrating information from the prior scans is notable and can be a key component of AI applications in lung cancer specifically and in oncologic imaging in general because much of the workflow in oncologic imaging includes the evaluation of serial scans over time in each patient. The models focusing on single timepoint analyses can address only a limited portion of the clinical questions. In the test dataset consisting of 6,716 cases (86 cancer-positives) from the National Lung Screening Trial (NLST), this model achieved an area under the receiver operating characteristic (AUC) of 94.4% for lung cancer risk prediction, which is considered to be a state-of-the-art performance. The model performed similarly with an AUC of 95.5% on an independent clinical validation set of 1,139 cases, demonstrating a step toward automated image evaluation for lung cancer risk estimation using AI.
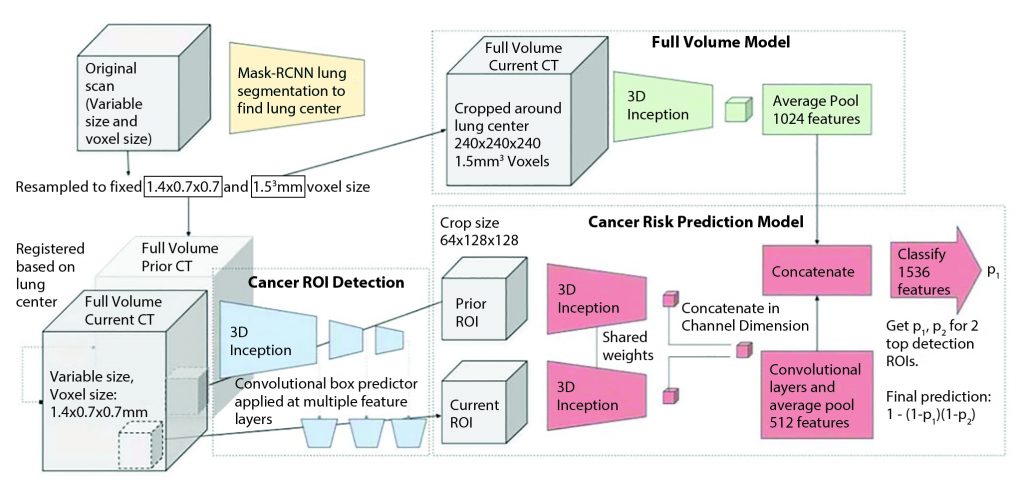
The model is trained to encompass the entire CT volume and automatically produce a score predicting the cancer diagnosis. In all cases, the input volume is first resampled into two different fixed voxel sizes as shown. Two ROI detections are used per input volume, from which features are extracted to arrive at per-ROI prediction scores via a fully connected neural network. The prior ROI is padded to all zeros when a prior is not available.
Reprinted by permission from Springer Nature: Springer Nature, Nature Medicine, End-to-end lung cancer screening with three-dimensional deep learning on low-dose chest computed tomography, Diego Ardila et al, 2019.
Furthermore, the two-part retrospective reader study was performed to compare the model performance with that of six U.S. board-certified radiologists. In the first part, each of the six radiologists and the model evaluated a subset of the test dataset consisting of 507 participants (83 of whom with cancer) from NLST, without access to previous CT scans. The patient demographics and clinical history were given to the radiologists but not to the model. The performance of all six radiologists trended at or below the model’s AUC of 95.9%. In the second part, the radiologists and the model evaluated current and previous year CT scans and interpreted 308 CT volumes from the cohort used in the first part of the comparison. Of note, these 308 scans were not from the initial baseline screening CT; rather, each scan had the previous year CT that was presented as a comparison. The model’s AUC was 92.6%, which was similar to the radiologists’ performance. Interestingly, both the model and the radiologists performed worse in this second part of the comparison compared with the first. Presumably this was because obviously malignant cases were diagnosed at the first screening and were not included in the cohort for the second part of the study, leaving more challenging evaluations for the second part. One of the goals of AI implementation is to reduce the workload for radiologists regarding straightforward evaluations, allowing them to spend increased time on complex cases. The results of the comparison indicate the need for further work to achieve this goal.
The study by Ardila et al. also commented on the fundamental questions raised by AI approaches, particularly its perceived “black box” nature, and provided deeper assessment of the modeling results for their validity. They also explored how the model evaluates risk of malignancy and found a few possible clues; however, many unknowns persist about the model’s prediction process, emphasizing that the transparency of deep-learning models is still at an early stage. This is another important message of this article, which honestly declares the unknown aspects of AI models and describes the attempts to open (or at least to peek into) the black box. Many of the AI studies simply describe their models’ performance out of the black box, without addressing the need for further examinations to decode how the outcomes are reached in the model. As stated by Ardila et al., such attempts are essential for physicians to take advantage of the features used by the model to achieve higher performance.
Detection and diagnosis are one of the areas that have been extensively studied for AI approaches in lung cancer.3-5 In addition to improving diagnostic accuracy, AI approaches have been used to improve reproducibility of quantitative feature extraction in radiomics of lung lesions.4 In a study of 104 patients with pulmonary nodules or masses, Choe et al.4 demonstrated that a CNN-based CT image conversion improved the reproducibility of CT radiomic features obtained using different reconstruction kernels. The study demonstrated another meaningful application of AI in quantitative tumor imaging of the lung4,5 because improving reproducibility is a critical step for quantitative imaging techniques, such as radiomics, to prove their practical value in the clinical setting. Imaging features derived from the AI approaches have also shown promise in describing prognostic and molecular phenotypes,6 which can be further investigated in the specific setting of lung cancer.
AI approaches have also been applied in the areas beyond radiologic imaging to address important questions for its detection, diagnosis, and prognostication of patients with cancer. For example, Mobadersanya et al.7 developed a survival prediction model using CNN that integrates information from histology images and genomic biomarkers into a unified prediction framework, resulting in superior prognostic accuracy compared to the current WHO paradigm in patients with brain tumors. Similar approaches can be explored in lung cancer, given an increasing trend for automated analyses of microscopic histology features.8 Finally, AI approaches based on deep natural language processing have been applied to the electronic health records in patients with cancer, allowing for speedy curation of relevant cancer outcomes.9
In conclusion, applying AI approaches to medicine has opened a number of new avenues for optimizing lung cancer detection, diagnosis, and prognostication. Along with the expanding applications of the AI models in various aspects of lung cancer, the attempts to understand the black box nature of the AI models are becoming particularly important so that physicians can also learn from the AI models that have been effectively trained—meaning that they have been created with sufficient volume and breadth of data—and can proceed to develop further sophisticated models to address truly challenging issues in lung cancer practice. ✦
About the Author: Dr. Nishino is Vice Chair of Faculty Development, Department of Imaging, Dana-Farber Cancer Institute, and Associate Professor of Radiology, Harvard Medical School.
References:
1. Ardila D, Kiraly AP, Bharadwaj S, et al. End-to-end lung cancer screening with three-dimensional deep learning on low-dose chest computed tomography. Nat Med. 2019;25(6):954-961.
2. Lundervold AS, Lundervold A. An overview of deep learning in medical imaging focusing on MRI. Z Med Phys. 2019;29(2):102-127.
3. Ciompi F, Chung K, van Riel SJ, et al. Towards automatic pulmonary nodule management in lung cancer screening with deep learning. Sci Rep. 2017;7:46479.
4. Choe J, Lee SM, Do KH, et al. Deep Learning-based Image Conversion of CT Reconstruction Kernels Improves Radiomics Reproducibility for Pulmonary Nodules or Masses. Radiology. 2019;292(2):365-373.
5. Park CM. Can Artificial Intelligence Fix the Reproducibility Problem of Radiomics? Radiology. 2019;292(2):374-375.
6. Lu H, Arshad M, Thornton A, et al. A mathematical- descriptor of tumor-mesoscopic-structure from computed-tomography images annotates prognostic- and molecular-phenotypes of epithelial ovarian cancer. Nat Commun. 2019;10(1):764.
7. Mobadersany P, Yousefi S, Amgad M, et al. Predicting cancer outcomes from histology and genomics using convolutional networks. Proc Natl Acad Sci U S A. 2018;115(13):E2970-E2979.
8. Yu KH, Zhang C, Berry GJ, et al. Predicting nonsmall cell lung cancer prognosis by fully automated microscopic pathology image features. Nat Commun. 2016;7: 12474.
9. Kehl KL, Elmarakeby H, Nishino M, et al. Assessment of Deep Natural Language Processing in Ascertaining Oncologic Outcomes From Radiology Reports. JAMA Oncol. 2019 Jul 25. [Epub ahead of print].